Building energy efficiency
- Jody Paterson
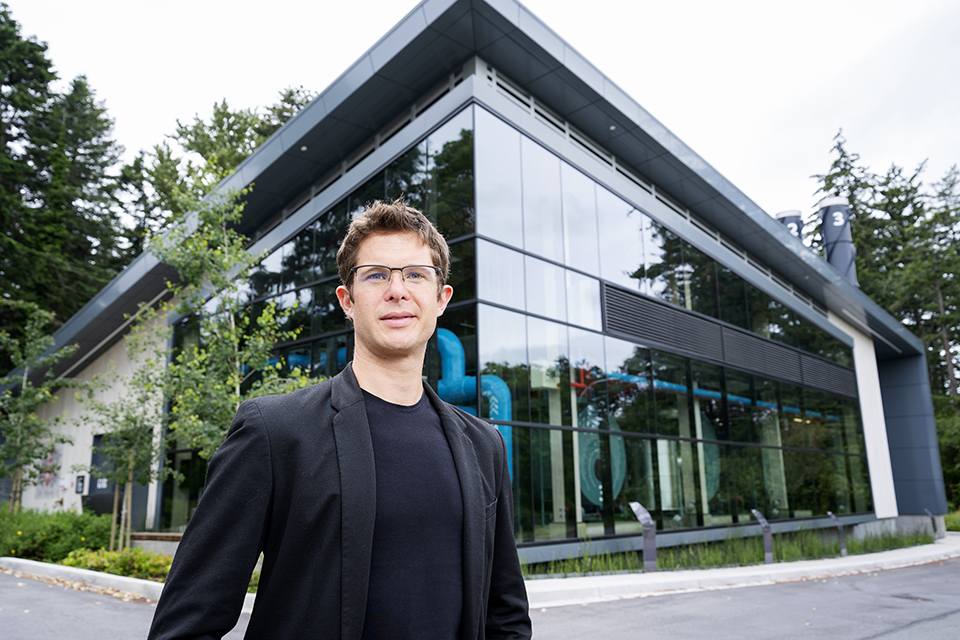
Machine learning helps researchers design energy-efficient buildings that don’t yet exist
Governments and industry are looking to university researchers for the tools to help them explore every aspect of building design through a lens of energy efficiency.
University of Victoria civil engineering professor Ralph Evins is helping lead this important work. Evins and his team are tapping into machine learning to create a modelling tool that can quickly calculate the energy efficiency of any possible design and how design choices interact with one another.
How much difference might bigger or smaller windows make? Thicker insulation? A different type of heating system? These are big questions when designing for energy efficiency, and differ for every situation and location, which are also factored into the model.
Current computer simulations are impossibly slow, hampering the creative nature of the design process. Evins’ industry-supported PhD research gave him an appreciation for the private sector’s pressing need for fast solutions. Computer simulations that take an hour or more to run are not a good fit for a professional needing to quickly test a multitude of design elements and materials to see how energy efficiency is affected.
Evins’ team has developed “surrogate” modelling that acts as a substitute for the more detailed computer models of physics-based processes that take much longer to run. Instead of long hours of waiting for computer calculations on myriad potential design elements, people using Evins’ model will be able to quickly and easily explore the trade-offs and interactions between design choices and performance metrics.
We’re putting better tools in place. Optimization for energy efficiency has been the buzzword since my PhD years, but this new modelling approach allows for the more intuitive kind of process that architects, engineers and developers use when they’re thinking about building design.
—Ralph Evins, UVic civil engineering professor
The result is a real-time tool that puts machine-learning algorithms to work solving more important world issues than how to connect social media users to more cat videos, says Evins with a smile.
The multi-disciplinary research is funded by the Natural Sciences and Engineering Research Council, the CANARIE Research Software Program and consulting engineering firm WSP—one of eight companies and 10 other entities helping review and steer the research.
The tools being developed will help these partners to meet the stringent new BC Energy Step Code, which incentivizes energy efficiency in new construction. Evins’ students bring a variety of backgrounds to the research, including extensive experience in the building industry that led them to join Evins in the search for better building models.
Evins believes the surrogate modelling can be equally useful for exploring retrofits for existing buildings. With big data sets now available to researchers, including anonymized data from 80,000 households with ecobee smart thermostats, the models can be used to explore when it makes more sense to retrofit a building than to replace it.
The modelling also helps industry prepare for a changing future. Evins works with another UVic entity, the Pacific Climate Impacts Consortium, to incorporate future climate modelling into the important work of designing buildings for new climate realities. Surrogate modelling tools for increased energy efficiency are critical components in helping Canada prepare for climate change and achieve its binding emissions reduction commitments.
EdgeWise
Machine learning involves putting computer algorithms to work finding patterns in massive quantities of data. It’s the driving force behind tech giants like Google, Amazon and Netflix, but has many more applications beyond shaping our shopping and surfing habits. Evins’ surrogate modelling work uses machine learning to understand building energy performance so that design professionals can quickly and easily assess different design elements.
Energy “optimization” has been a hot topic for university researchers for more than a decade, but has had little traction in industry. Surrogate modelling provides an opportunity for building designers to achieve optimal designs while working in their usual intuitive way. “It’s very hard to sit down with someone and say, ‘List everything you want in a building,’ which is how optimization works. With surrogate modelling, it isn’t necessary to state the problem like that,” notes Evins.
The data generated for Evins’ surrogate modelling tools is revealing information that no one has seen before. The work is bringing unprecedented insight into the intricate relationship between individual design elements and how that interplay affects energy efficiency.
Surrogate modelling is multi-disciplinary. Evins’ team features researchers with backgrounds in mathematics, physics, computer science, architecture and engineering.
Evins’ background is in fluid dynamics, which could prove useful as his research team expands its surrogate modelling work to encompass how air flow in and around buildings affects energy efficiency.
Photos
In this story
Keywords: computers, clean energy, industry partnerships, mathematics, physics, research, technology, ECS expansion
People: Ralph Evins, Pacific Climate Impacts Consortium
Publication: knowlEDGE