Expert Q&A on mathematical modelling of COVID-19
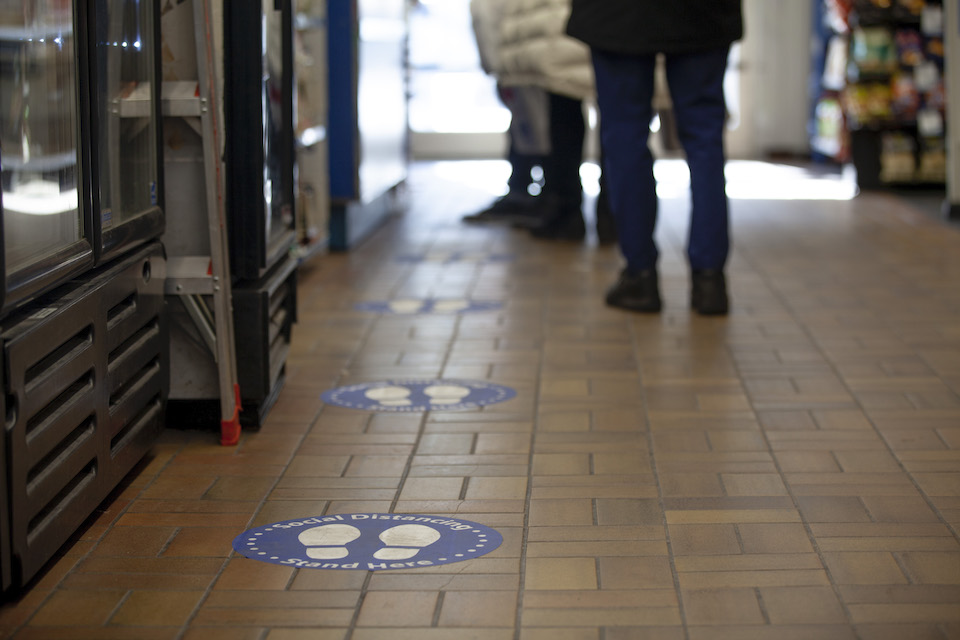
Over the past weeks and months, physical distancing, self-quarantine and self-isolation measures have been enacted over much of the globe, but we are still learning how effective these strategies are in slowing the spread of COVID-19.
In order to measure the effectiveness of these contact-reduction strategies and further refine them, we turn to mathematical and computer simulation models like the ones being developed by University of Victoria statistical mathematician Junling Ma.
His project is part of a wide-ranging collaboration between the Fields Institute, Pacific Institute for the Mathematical Sciences, and Centre de Recherches Mathématiques to engage in all aspects of the mathematical modelling of COVID-19. It is funded by the Canadian Institutes of Health Research (CIHR) Canadian 2019 Novel Coronavirus (COVID-19) Rapid Research Funding Opportunity.
Q Your CIHR-funded project will create a modelling tool. How will it work?
A. The tool we create will be able to produce more precise predictions of the outcome of the outbreak by considering realistic contact patterns of the population, given current social-distancing measures. This will allow policymakers to evaluate and compare the effectiveness of measures.
Q Are there particular challenges to modelling COVID-19 compared to diseases like influenza?
A. COVID-19 can transmit asymptomatically and is very contagious, creating uncertainty in some of the key parameters we typically use in disease modelling. Without being aware that they have symptoms, a patient can transmit COVID-19 to another person through just a cough or sneeze. This creates a challenge in determining how long it takes a patient to become infectious or how long they keep infecting others. Since we can’t directly observe this, we have to use other parameters, such as generation time, to go around this uncertainty.
Q What is generation time?
A. It’s the interval of time between when patients become infected and when they subsequently infect others. It measures specific infections rather than a theoretical state of infectiousness. Through contact tracing, we know details about when patients have been infected and when they infected other people. We can use this information to build more accurate models of the spread of COVID-19.
Q Mathematical modelling terms, such as “flattening the curve,” “reaching peak” and “exponential growth” have become a part of daily conversation in recent weeks. Is this important?
A. In the current situation, modelling is a crucial tool for policymakers and for the public to know what could happen in the future. I’m happy to see these terms getting wider acceptance. It shows that people are paying attention and putting trust into mathematical knowledge.
Q Is there a risk in using modelling terms in everyday conversation?
A. I think modellers need to be aware of the messages we are conveying and do a better job of explaining terminology, as it can have important implications into how people understand diseases.For example, the term reaching peak might lead someone to believe that once a population reaches peak, the number of infections will continually decrease. While that’s true for a disease that has infected a big fraction of the population, like the seasonal flu, diseases can have multiple peaks if the first peak is caused by control measures. For example, the 2003 SARS outbreak in Toronto had two peaks.
With COVID-19, where only a very small fraction of the population has been infected, the trajectory may have multiple peaks, depending on the population’s behaviour. If people think that a disease has only one peak, it could cause them to be less cautious or not respect physical-distancing measures.
Q Are you doing any other research on COVID-19?
A. I’m wrapping up related research measuring the generation time of COVID-19, based on case studies from China, excluding Hubei province. Of note is our finding that the 14-day quarantine period that is currently recommended may not be long enough to stop the importation of COVID-19 cases and has a sizeable failure rate. We expect to report more on these results soon. I’m also working on another project estimating the number of unreported cases locally.
Q What are the limitations of mathematical models?
A. Mathematical models are important, but they make assumptions. Some are suitable for some situations, but not others. Models don’t always reflect what is going to happen, but are designed for different scenarios and are based on assumptions. All models have their limitations, but they are useful for the scenario that they are designed for.Photos
Media contacts
Junling Ma (Dept. of Mathematics and Statistics) at 250-721-7442 or junlingm@uvic.ca
Vimala Jeevanandam (Communications Officer, Science) at 250-721-8745 or scieco@uvic.ca
Jennifer Kwan (University Communications + Marketing) at 250-721-7641 or researchcomm@uvic.ca
In this story
Keywords: COVID, health, mathematics, research, viruses, mathematics & statistics, Pacific Institute for the Mathematical Sciences
People: Junling Ma